A Busy Summer of Presentations for AMISTAD Lab
September 27, 2022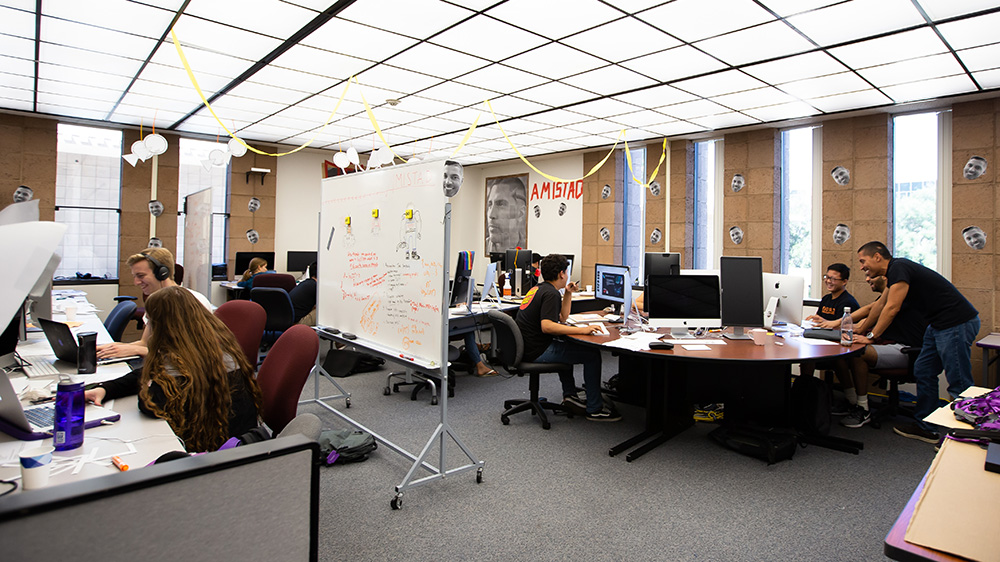
In July 2022, AMISTAD lab sent two students, Nico Espinosa Dice ’22 and Ramya Ramalingam ’21, to present their research on information-theoretic generalization bounds for supervised learning at the 2022 International Joint Conference on Neural Networks, in Padua, Italy. Sponsored by Google and Nvidia, the conference was hosted by the International Neural Networks Society and the University of Padua, the fifth oldest surviving university in the world, where Copernicus was once a student and Galileo was the former chair of mathematics.
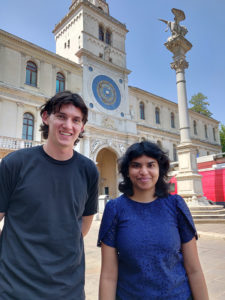
The students presented their paper, “Bounding Generalization Error Through Bias and Capacity” (co-authored with Megan Kaye ’22 and CS professor George Montañez) to an international audience of university students and professors. In the paper, they use recent theoretical work in the machine learning literature to derive bounds on how poorly a learning algorithm can perform on new examples it hasn’t seen in training. Guided by AMISTAD’s belief that algorithms with excess capacity for memorization will act as rote learners and fail to generalize to new examples, they proved that low capacity directly bounds future generalization error. This work is the first to introduce generalization bounds within the Algorithmic Search Framework, a formal system for understanding machine learning and AI as a type of feedback-informed search process.
Dice is an incoming PhD student at Cornell CS who will spend a deferral year at a cryptocurrency startup and Ramalingam is a CS PhD student at the University of Pennsylvania. The presentation was generously supported by Leeds Student Conference Funding, HMC’s mathematics and computer science departments, and faculty start-up funding.
- Paper: Ramalingam R, Espinosa Dice N, Kaye M, Montañez G, “Bounding Generalization Error Through Bias and Capacity.” 2022 International Joint Conference on Neural Networks (IJCNN 2022), July 18-22, Padua, Italy.
- Abstract: We derive generalization bounds on learning algorithms through algorithm capacity and a vector representation of inductive bias. Leveraging the algorithmic search framework, a formalism for casting machine learning as a type of search, we present a unified interpretation of the upper bounds of generalization error in terms of a vector representation of bias and the mutual information between the hypothesis and the dataset.
On Aug. 2, William Yik ’24 and Rui-Jie Yew ’21 Scripps (AMISTAD alumna and now a master’s student at MIT CS) presented research to a large crowd of almost 200 researchers from around the world at the AAAI/ACM Conference on Artificial Intelligence, Ethics, and Society. The conference, organized by AAAI, ACM, and ACM SIGAI, was held at Oxford University, Keble College, one of the world’s oldest and most prestigious universities. Conference sponsors included the NSF, Google, Meta, Sony and IBM.
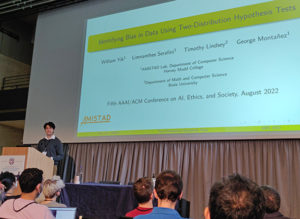
The focus of the conference was ethical and societal issues of AI systems, and both papers found responsive audiences. Yik’s paper was focused on the issue of bias in training data, and developing ways to spot it. His work was done as part of summer research during 2021, along with student coauthors Limnanthes Serafini ’24 and Timothy Lindsey of Biola University and Prof. George Montañez. The paper presents novel statistical hypothesis tests to rule out whether the source of a dataset could plausibly be considered unbiased, using easy-to-specify examples of what unbiased generation would look like. This approach differs from typical work in the field, which often checks for fairness in machine learning system outputs rather than their inputs. Yew’s work presented a public policy approach to harm mitigation in AI systems, using ideas inspired by existing contract law.
Yik’s paper (with accompanying short video); with a layperson’s introduction to the work.
- Citation: Identifying Bias in Data Using Two-Distribution Hypothesis Tests
William Yik, Limnanthes Serafini, Timothy Lindsey, and George D. Montañez
AIES ’22: Proceedings of the 2022 AAAI/ACM Conference on AI, Ethics, and Society,
July 2022. Pages 831–844. https://doi.org/10.1145/3514094.3534169 - Abstract: As machine learning models become more widely used in important decision-making processes, the need for identifying and mitigating potential sources of bias has increased substantially. Using two-distribution (specified complexity) hypothesis tests, we identify biases in training data with respect to proposed distributions and without the need to train a model, distinguishing our methods from common output-based fairness tests. Furthermore, our methods allow us to return a “closest plausible explanation” for a given dataset, potentially revealing underlying biases in the processes that generated them. We also show that a binomial variation of this hypothesis test could be used to identify bias in certain directions, or towards certain outcomes, and again return a closest plausible explanation. The benefits of this binomial variation are compared with other hypothesis tests, including the exact binomial. Lastly, potential industrial applications of our methods are shown using two real-world datasets.